White Papers
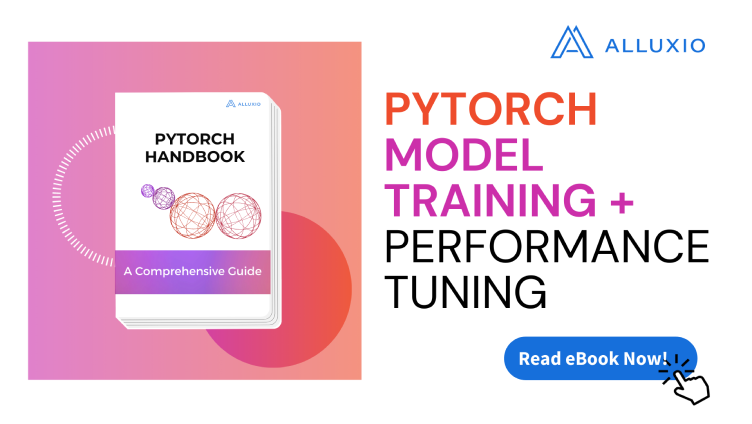
Introduction
The exponential growth of the raw computational power, communication bandwidth, and storage capacity results in continuous innovation in how data is processed and stored. To address the evolving nature of the compute and storage landscape, we are continuously advancing Alluxio, a state-of-the-art memory-centric virtual distributed storage system.
This blog post highlights unified namespace, an abstraction that makes it possible to access multiple independent storage systems through the same namespace and interface. With Alluxio’s unified namespace, applications simply communicate with Alluxio and Alluxio manages the communication with the different underlying storage systems on applications’ behalf.
In the remainder of this blog post, we first discuss the importance of decoupling computation from data, providing motivation for Alluxio’s unified namespace. We then describe the feature itself and present two examples that illustrate how unified namespace makes it possible for applications to transparently work with different storage systems.
Benefits
Leveraging Alluxio’s unified namespace provides the following benefits:
- Future-proofing your applications: applications can communicate with different storage systems, both existing and new, using the same namespace and interface; seamless integration between applications and new storage systems enables faster innovation
- Enabling new workloads: integrating an application or a storage system with Alluxio is a one-time effort which enables the application to access many different types of storage systems and the storage system to be accessed by many different types of applications
Motivation
There are two common approaches to large-scale data processing. Computation and data can be either co-located in the same cluster or dedicated compute and storage clusters are used. On one hand, managing compute and storage resources separately enables scaling infrastructure resources in a cost-effective manner. On the other hand, co-locating computation and data avoids expensive data transfers, which benefits I/O intensive workloads such as data processing pipelines.
In a previous blog post, we talked about how to use Alluxio with Spark and demonstrated the performance benefits Alluxio provides when Spark communicates with remote storage such as S3. Alluxio realizes these performance benefits through its state-of-the-art memory-centric storage layer, possibly improving application performance by orders of magnitude.
However, decoupling computation from data at the physical level does not address the logical dependencies between computation and data. In particular, because of the differences between different types of applications and storage systems, it can be challenging to allow applications to access data across different types of storage systems, both existing and new.
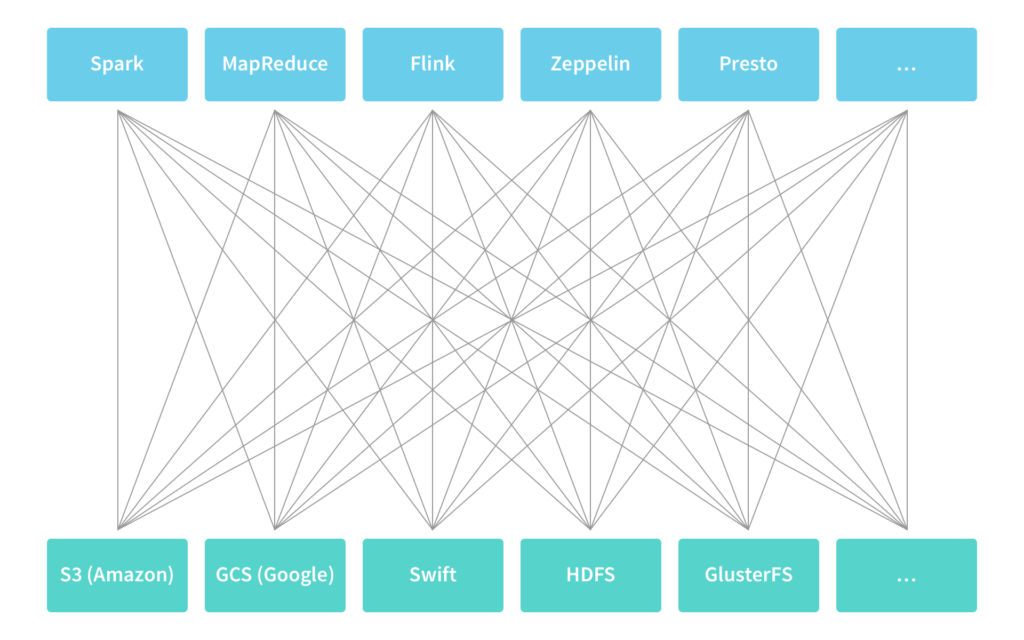
Common solutions to this problem are sub-optimal. Applications either need to be: 1) integrated with different types of storage systems, which does not scale as the number of applications and storage systems grows; or 2) data needs to be first extracted to a temporary location that applications know how to access, which leads to data duplication and increases time to insight.
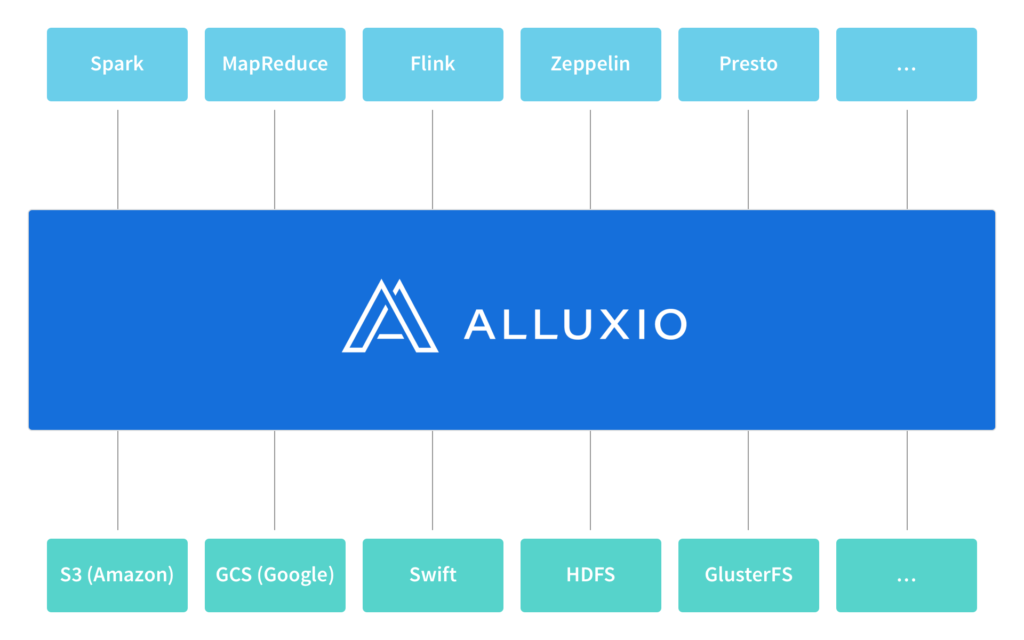
In this blog post, we talk about how Alluxio addresses this challenge by providing a layer of indirection called unified namespace that logically decouples the computation from data. With Alluxio’s unified namespace, applications simply communicate with Alluxio while Alluxio handles the communication with the different underlying storage systems on applications’ behalf.
Unified Namespace
Storing, accessing, and managing data at scale is an increasingly common challenge, rooted in the fact that data typically spans multiple storage systems with different interfaces and independent namespaces. To address this challenge, Alluxio provides a unified namespace, a feature that greatly simplifies data management at scale by making it possible to interact with different storage systems using the same namespace and interface.
Similar to how a local workstation allows applications to use the same interface to access different devices (such hard disks or USB drives), Alluxio allows distributed applications to use the same interface to access different types of distributed storage systems (such as S3 or HDFS).
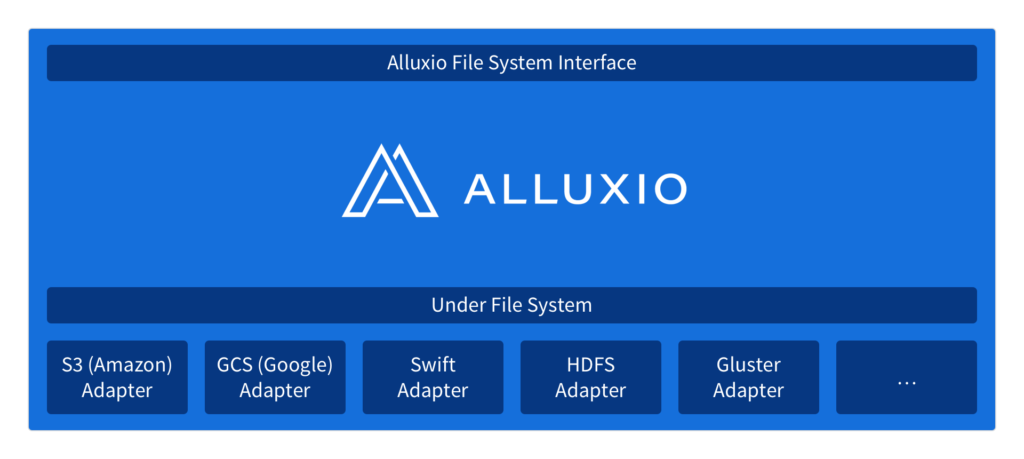
However, Alluxio’s unified namespace is not just an interface; it is also a set of adapters that make it possible to use the same interface to access many popular storage systems. In addition, accessing data through Alluxio leverages Alluxio’s memory-centric data management layer, enabling significant performance benefits.
Similar to how a personal computer maps different local paths to different devices, Alluxio maps different Alluxio paths to different underlying storage systems. The mapping is dynamic and Alluxio provides an API for creating and removing these mappings and transparently surfacing objects from the underlying storage system in Alluxio.
Examples
In this section, we walk through a couple examples to illustrate how Alluxio’s unified namespace works in the real world.
Working With Multiple Storage Systems
In this example, we illustrate how Alluxio’s unified namespace enables applications to use one API to interact with multiple different storage systems at the same time.
For the sake of this example, we assume that the application is written in Java and is sharing data between HDFS and S3.
The following logic illustrates how Alluxio can be used to read data from HDFS, process it, and then write the results to S3:
package io;
import
alluxio.client.file.FileInStream;
import
alluxio.client.file.FileOutStream;
import
alluxio.client.file.FileSystem;
// mount
HDFS and S3 to Alluxio
FileSystem fileSystem = FileSystem.Factory.get();
fileSystem.mount("/mnt/hdfs", "hdfs://...");
fileSystem.mount("/mnt/s3", "s3n://...");
// read
data from HDFS
AlluxioURI inputUri = new AlluxioURI("/mnt/hdfs/input.data");
FileInStream is = fileSystem.openFile(inputUri);
... // read
data
is.close();
... // perform computation
// write data to S3
AlluxioURI outputUri = new AlluxioURI("/mnt/s3/output.data");
FileOutStream os = fileSystem.createFile(outputUri);
... // write data
os.close();
A special case of the above example is the following logic, which can be used to copy data from one storage system to another.
package io;
import
alluxio.client.file.FileInStream;
import
alluxio.client.file.FileOutStream;
import
alluxio.client.file.FileSystem;
import
org.apache.commons.io.IOUtils;
// mount
HDFS and S3 to Alluxio
FileSystem fileSystem = FileSystem.Factory.get();
fileSystem.mount("/mnt/hdfs", "hdfs://...")
fileSystem.mount("/mnt/s3", "s3n://...")
// copy data from HDFS to S3
AlluxioURI inputUri = new AlluxioURI("/mnt/hdfs/input.data");
AlluxioURI outputUri = new AlluxioURI("/mnt/s3/output.data");
FileInStream is = fileSystem.openFile(inputUri);
FileOutStream os = fileSystem.createFile(outputUri);
IOUtils.copy(is, os);
is.close();
os.close();
A code-complete version of this example can be found here.
Porting an Application to a Different Storage System
In this example, we illustrate how using unified namespace greatly reduces the effort needed to port an application when the data the application accesses moves to a different storage system.
For the sake of this example, let us again assume that the application is written in Java and that the data it accesses moves from HDFS to S3.
Without Alluxio, the original application would use the HDFS API for read and writing the data:
package io;
import
org.apache.hadoop.conf.Configuration;
import
org.apache.hadoop.fs.FSDataInputStream;
import
org.apache.hadoop.fs.FSDataOutputStream;
import
org.apache.hadoop.fs.FileSystem;
import
org.apache.hadoop.fs.Path;
// reading data
Path path = new Path("hdfs://<host>:<port>/<path>");
FileSystem fileSystem = path.getFileSystem(new Configuration());
FSDataInputStream is = fileSystem.open(path);
... // read
data
is.close();
... // perform computation
// writing data
Path path = new Path("hdfs://<host>:<port>/<path>");
FileSystem fileSystem = path.getFileSystem(new Configuration());
FSDataOutputStream os = fileSystem.create(path);
... // write data
os.close();
When the data moves to S3, the application needs to use a different API, such as jets3t, to read and write the data:
package io;
import
org.jets3t.service.impl.rest.httpclient.RestS3Service;
import
org.jets3t.service.model.S3Object;
import
org.jets3t.service.security.AWSCredentials;
import
java.io.InputStream;
// reading data
RestS3Service client = new RestS3Service();
S3object object = client.getObject(<bucket>, <key>);
InputStream is = object.getDataInputStream();
... // read
data
is.close();
... // perform computation
// writing data
OutputStream os = new FileOutputStream();
... // write data
os.close();
InputStream is = new FileInputStream(<tmpFile>);
RestS3Service client = new RestS3Service(<credentials>);
S3Object object = new S3Object(<key>);
object.setDataInputStream(is);
client.putObject(<bucket>, object);
is.close();
In addition to the difference in the above logic, the logic for reading and writing data (not shown) needs to change as well to reflect the differences between HDFS API and S3 API.
In contrast to the above changes, with Alluxio, the application can use the following logic to access data from both HDFS and S3:
package io;
import
alluxio.client.file.FileInStream;
import
alluxio.client.file.FileOutStream;
import
alluxio.client.file.FileSystem;
// mount
a storage system (HDFS or S3) to Alluxio
FileSystem fileSystem = FileSystem.Factory.get();
fileSystem.mount("/mnt/data", <url>;)
// reading data
AlluxioURI uri = new AlluxioURI("/mnt/data/...");
FileInStream is = fileSystem.openFile(uri);
... // read
data
is.close();
... // perform computation
// writing data
AlluxioURI uri = new AlluxioURI("/mnt/data/...");
FileOutStream os = fileSystem.createFile(uri);
... // write data
os.close();
In particular, when data moves from HDFS to S3, the only part of the application that needs to be updated is the <url> to mount, which can be read from configuration or a command-line argument to avoid any application changes at all.
Conclusion
Alluxio’s unified namespace provides a layer of indirection that logically decouples the computation from data. Applications simply communicate with Alluxio while Alluxio handles the communication with the different underlying storage systems on applications’ behalf.
Alluxio supports many popular storage systems including S3, GCS, Swift, HDFS, OSS, GlusterFS, and NFS. Adding support for new storage systems is relatively straightforward — the existing adapters are less than a thousand lines of code each.
Please do not hesitate to contact us if you would like to propose or lead an integration. We welcome more integrations and look forward to hearing from you!
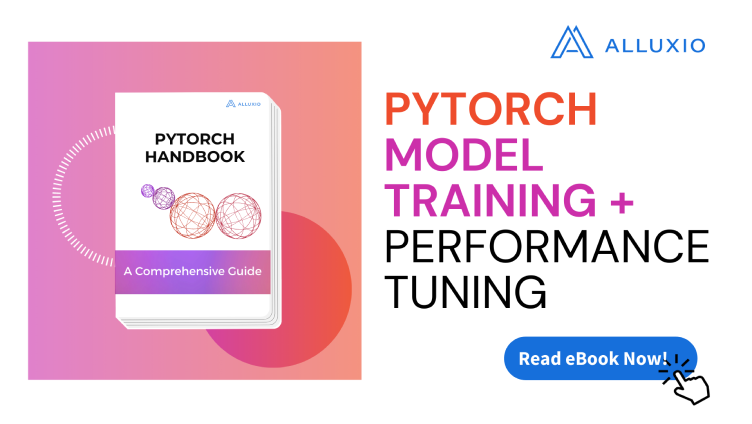
For business to not just survive — but to flourish — it’s become imperative to make decisions with near immediacy, continuously pivot strategy and tactics, and merge streams of inquiries into meaningful action. Executing requires high-frequency insights — the competitive advantage in today’s frenetic business landscape. Together with Alluxio, Inc., we enable businesses to gain the competitive advantage with faster time to insights with our integrated solution of Cray high-performance analytics platform and Alluxio’s memory-speed virtual storage system — Alluxio Enterprise Edition.
For businesses on the cusp of innovation and seeking that information advantage, Cray has fused supercomputing with an open, standards-based framework to deliver an industry first: the Cray® Urika®-GX agile analytics platform. This advanced platform has an unprecedented combination of versatility and speed to tackle the largest problems at super scale and uncover hidden patterns with a fast time to insight.
Alluxio provides a unified view of enterprise data that spans disparate storage systems, locations and clouds, allowing any big data compute framework to access stored data at memory speed. Alluxio Enterprise Edition gives Cray customers the ability to co-locate compute and data with memory-speed access to data while virtualizing across different storage systems under a unified namespace. Alluxio Enterprise Edition supercharges data storage and gives customers an easy-to-install integrated package, with an administrative interface as well as mission-critical features such as enterprise security, data replication and enterprise-grade support and professional services.

Alluxio proves its value in production scenarios where customers need to analyze vast amounts of data in real-time. It overcomes I/O limitations for running big data workloads that access remote storage systems and allows big data workloads to share data at memory speed for orders of magnitude higher performance in running jobs.
Benefits of the Urika-GX platform and Alluxio Enterprise Edition integration
Easy to deploy for access to multiple storage types
With this integration, you can deploy Alluxio in minutes and realize fast on-demand analytics by unified in-memory access to a multitude of data sources such as Ceph, HDFS, Amazon S3 and Google Cloud Storage. Choose between command-line and GUI-based installation to suit your preferences on any number of high-memory compute nodes on the Urika-GX platform.
The Urika-GX system is optimized for Apache Spark™ in-memory analytics, and combining that with Alluxio on Urika-GX gives you single data access gateway. You can expand this in-memory computation capabilities to other frameworks with Alluxio on the Urika-GX platform.
Unified view and management of all your data
Alluxio connects any compute framework, such as Spark or Presto, to disparate storage systems via a unified global file system namespace, and enables any application to interact with data stored on premise and in the cloud at memory speed. This eliminates data duplication and migration, as well as enables new workflows across data stored in any storage system, including cross-data center storage.
Efficient resource utilization
By decoupling compute from storage, Alluxio allows organizations to scale compute and storage resources independently. You can scale number of compute nodes with high-performance and reliable components and leave the job of data access to Alluxio on the Urika-GX platform, which utilizes Cray’s supercomputing network for node-to-node communication, thus ensuring effective “data-locality” even for remote data sources.
High-performance and predictable SLA
Alluxio is co-located with compute, and thereby provides memory-speed access to data, and orders of magnitude improvement in runtime for different types of jobs. The Urika-GX system, with its dense memory hierarchy, enables Alluxio to cache large amounts of multiple data types and sources to enable fast data access, high computational performance and predictable SLAs.
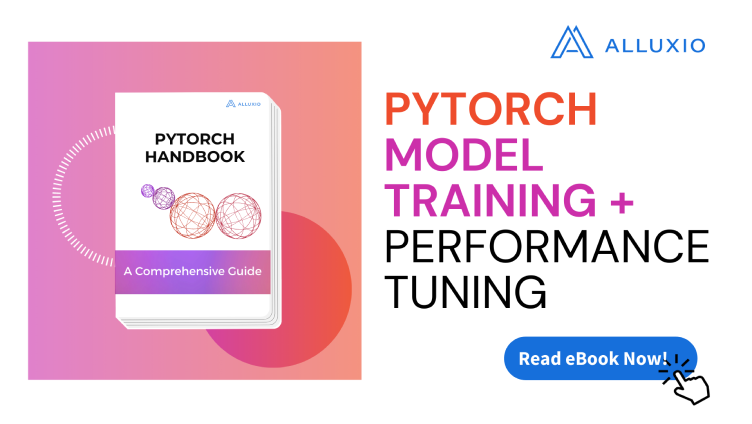
Introduction
Many organizations deploy Alluxio together with Spark for performance gains and data manageability benefits. Qunar recently deployed Alluxio in production, and their Spark streaming jobs sped up by 15x on average and up to 300x during peak times. They noticed that some Spark jobs would slow down or would not finish, but with Alluxio, those jobs could finish quickly. In this blog post, we investigate how Alluxio helps Spark be more effective. Alluxio increases performance of Spark jobs, helps Spark jobs perform more predictably, and enables multiple Spark jobs to share the same data from memory. Previously, we investigated how Alluxio is used for Spark RDDs. In this article, we investigate how to effectively use Spark DataFrames with Alluxio.
Alluxio and Spark CachING
Storing Spark DataFrames in Alluxio memory is very simple, and only requires saving the DataFrame as a file to Alluxio. This is very simple with the Spark DataFrame write
API. DataFrames are commonly written as parquet files, with df.write.parquet()
. After the parquet is written to Alluxio, it can be read from memory by using sqlContext.read.parquet()
.
In order to understand how saving DataFrames to Alluxio compares with using Spark cache, we ran a few simple experiments. We used a single worker Amazon EC2 r3.2xlarge instance, with 61 GB of memory, and 8 cores. We used Spark 2.0.0 and Alluxio 1.2.0 with the default configurations. We ran both Spark and Alluxio in standalone mode on the node. For the experiment, we tried different ways of caching Spark DataFrames, and saving DataFrames in Alluxio, and measured how the various techniques affect performance. We also varied the size of the data to show how data size affects performance.
Saving DataFrames
Spark DataFrames can be “saved” or “cached” in Spark memory with the persist()
API. The persist()
API allows saving the DataFrame to different storage mediums. For the experiments, the following Spark storage levels are used:
MEMORY_ONLY
: stores Java objects in the Spark JVM memoryMEMORY_ONLY_SER
: stores serialized java objects in the Spark JVM memoryDISK_ONLY
: stores the data on the local disk
Here is an example of how to cache a DataFrame with the persist()
API:
df.persist(MEMORY_ONLY)
An alternative way to save DataFrames to memory is to write the DataFrame as files in Alluxio. Spark supports writing DataFrames to several different file formats, but for these experiments we write DataFrames as parquet files. Here is an example of how to write a DataFrame to Alluxio memory:
df.write.parquet(alluxioFile)
Querying “saved” DataFrames in Alluxio
After DataFrames are saved, either in Spark or Alluxio, applications can read them to computations. In our experiments, we created a sample DataFrame with 2 float columns, and the computation was a sum on both columns.
When the DataFrame is stored in Alluxio, to read the data in Spark is as simple as reading the file from Alluxio. Here is an example of reading our sample DataFrame in Alluxio.
df
= sqlContext.read.parquet(alluxioFile)
df.agg(sum("s1"), sum("s2")).show()
We performed this aggregation on the DataFrame from Alluxio parquet files, and from various Spark persist storage levels, and we measured the time it took for the aggregation. The figure below shows the completion times for the aggregations.

The figure shows that performing the aggregation on the DataFrame read from the Alluxio parquet file results in very predictable and stable performance. However, when reading DataFrames from the Spark cache, the performance is high for small data sizes, but larger data sizes significantly hurts the performance. For the various Spark storage levels, after about 20GB of input data, the aggregation slows down and increases significantly.
With Alluxio memory, the DataFrame aggregation performance is slightly slower than with Spark memory for smaller data sizes, but as the data size grows, reading from Alluxio performance significantly better as it scales linearly with the data size. Since the performance scales linearly, applications can process larger data sizes at memory speeds with Alluxio.
Sharing “saved” DataFrames with Alluxio
Alluxio also enables the ability to share data in-memory, even across different Spark jobs. After a file is written to Alluxio, that same file can be shared across different jobs, contexts, and even frameworks, via Alluxio’s memory. Therefore, if a DataFrame in Alluxio is frequently accessed by many applications, all the applications can read the data from the in-memory Alluxio file, and do not have to recompute it or fetch it from an external source.
To demonstrate the in-memory sharing benefits of Alluxio, we computed the same DataFrame aggregation in the same environment as described above. With the 50GB data size, we ran the aggregation in a separate Spark application, and measured the time it took to perform the computation. Without Alluxio, the Spark application must read the data from the source, which is the local SSD in this experiment. However, when using Spark with Alluxio, reading the data means reading it from Alluxio memory. Below are the results of the completion time for the aggregation.
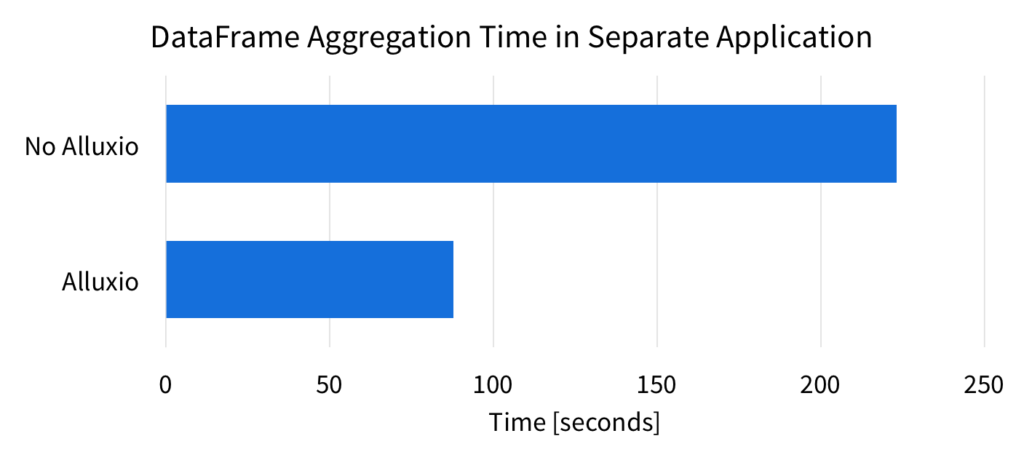
Without Alluxio, Spark has to read the data from the source again (local SSD). Reading from Alluxio is faster since the data is read from memory. With Alluxio, the aggregation is over 2.5x faster.
In the previous experiment, the source of the data was the local SSD. However, if the source of the DataFrame is slower or less predictable, the benefits of Alluxio is more significant. For example, Amazon S3 is a popular system for storing large amounts of data. Below are the results for when the source of the DataFrame is from Amazon S3.

The figure shows the average aggregation completion time over 7 runs. The error bars in the figure represent the min and max range of the completion times. These results clearly show, that Alluxio significantly improves the average performance of the computation. This is because with Alluxio, Spark can read the DataFrame directly from Alluxio memory, instead of fetching the data from S3 again. On average, Alluxio speeds up the DataFrame computation over 10x.
Since the source of the data is Amazon S3, Spark without Alluxio has to fetch the data over the network, and this can result in unpredictable performance. This unstable performance is evident from the error bars of the figure. Without Alluxio, the Spark job completion times widely vary, by over 1100 seconds. With Alluxio, the completion times only vary by 10 seconds. Alluxio reduces the unpredictability by over 100x!
Because of the S3 network unpredictability, the slowest Spark run without Alluxio can take as long as over 1700 seconds, almost twice as slow as the average. On the other hand, the slowest Spark run with Alluxio is around 6 second slower than the average time. By considering the slowest runs, Alluxio speeds up the DataFrame aggregation by over 17x.
Conclusion
Alluxio helps Spark be more effective by enabling several benefits. This blog demonstrates how to use Alluxio with Spark DataFrames, and presents performance evaluations of the benefits.
- Alluxio can keep larger data sizes in memory to speed up Spark applications
- Alluxio enable sharing of data in-memory
- Alluxio provides stable and predictable performance
.png)
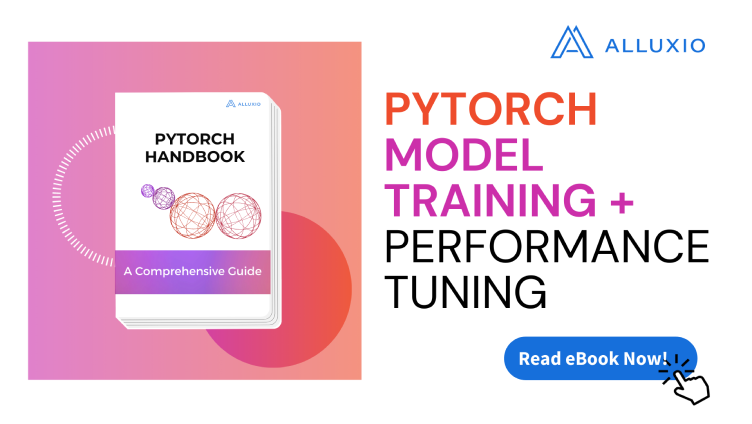
Introduction
The exponential growth of the raw computational power, communication bandwidth, and storage capacity results in continuous innovation in how data is processed and stored. To address the evolving nature of the compute and storage landscape, we are continuously advancing Alluxio, a state-of-the-art memory-centric virtual distributed storage system.
This blog post highlights unified namespace, an abstraction that makes it possible to access multiple independent storage systems through the same namespace and interface. With Alluxio’s unified namespace, applications simply communicate with Alluxio and Alluxio manages the communication with the different underlying storage systems on applications’ behalf.
In the remainder of this blog post, we first discuss the importance of decoupling computation from data, providing motivation for Alluxio’s unified namespace. We then describe the feature itself and present two examples that illustrate how unified namespace makes it possible for applications to transparently work with different storage systems.
Benefits
Leveraging Alluxio’s unified namespace provides the following benefits:
- Future-proofing your applications: applications can communicate with different storage systems, both existing and new, using the same namespace and interface; seamless integration between applications and new storage systems enables faster innovation
- Enabling new workloads: integrating an application or a storage system with Alluxio is a one-time effort which enables the application to access many different types of storage systems and the storage system to be accessed by many different types of applications
Motivation
There are two common approaches to large-scale data processing. Computation and data can be either co-located in the same cluster or dedicated compute and storage clusters are used. On one hand, managing compute and storage resources separately enables scaling infrastructure resources in a cost-effective manner. On the other hand, co-locating computation and data avoids expensive data transfers, which benefits I/O intensive workloads such as data processing pipelines.
In a previous blog post, we talked about how to use Alluxio with Spark and demonstrated the performance benefits Alluxio provides when Spark communicates with remote storage such as S3. Alluxio realizes these performance benefits through its state-of-the-art memory-centric storage layer, possibly improving application performance by orders of magnitude.
However, decoupling computation from data at the physical level does not address the logical dependencies between computation and data. In particular, because of the differences between different types of applications and storage systems, it can be challenging to allow applications to access data across different types of storage systems, both existing and new.
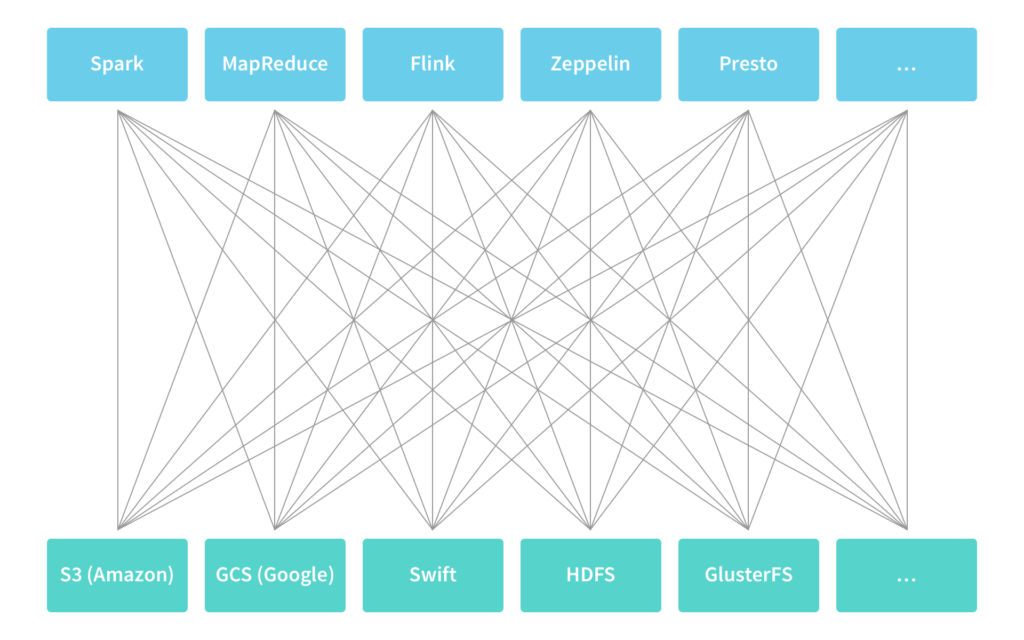
Common solutions to this problem are sub-optimal. Applications either need to be: 1) integrated with different types of storage systems, which does not scale as the number of applications and storage systems grows; or 2) data needs to be first extracted to a temporary location that applications know how to access, which leads to data duplication and increases time to insight.
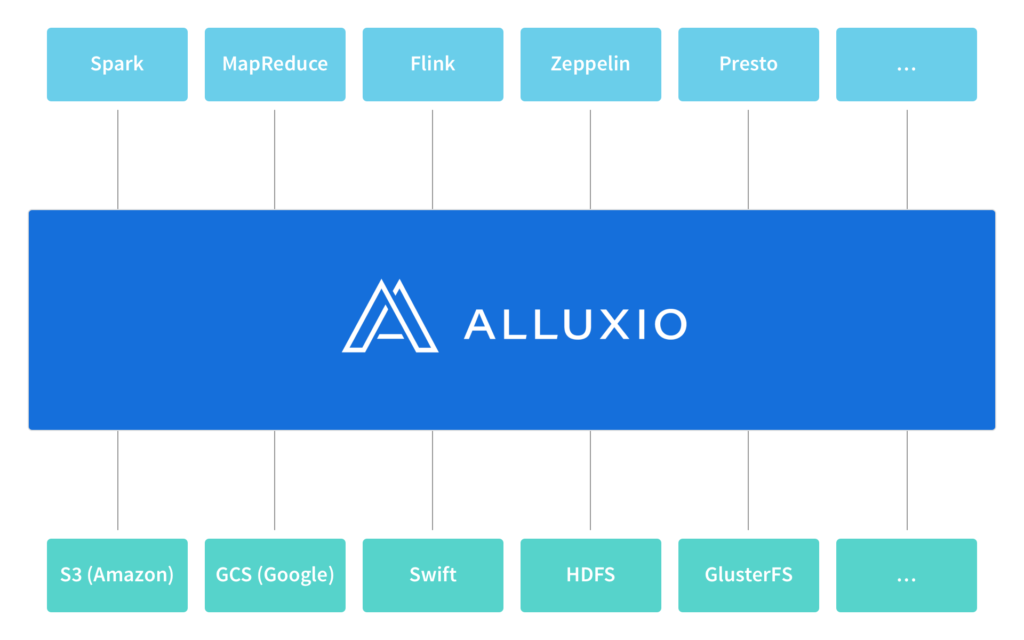
In this blog post, we talk about how Alluxio addresses this challenge by providing a layer of indirection called unified namespace that logically decouples the computation from data. With Alluxio’s unified namespace, applications simply communicate with Alluxio while Alluxio handles the communication with the different underlying storage systems on applications’ behalf.
Unified Namespace
Storing, accessing, and managing data at scale is an increasingly common challenge, rooted in the fact that data typically spans multiple storage systems with different interfaces and independent namespaces. To address this challenge, Alluxio provides a unified namespace, a feature that greatly simplifies data management at scale by making it possible to interact with different storage systems using the same namespace and interface.
Similar to how a local workstation allows applications to use the same interface to access different devices (such hard disks or USB drives), Alluxio allows distributed applications to use the same interface to access different types of distributed storage systems (such as S3 or HDFS).
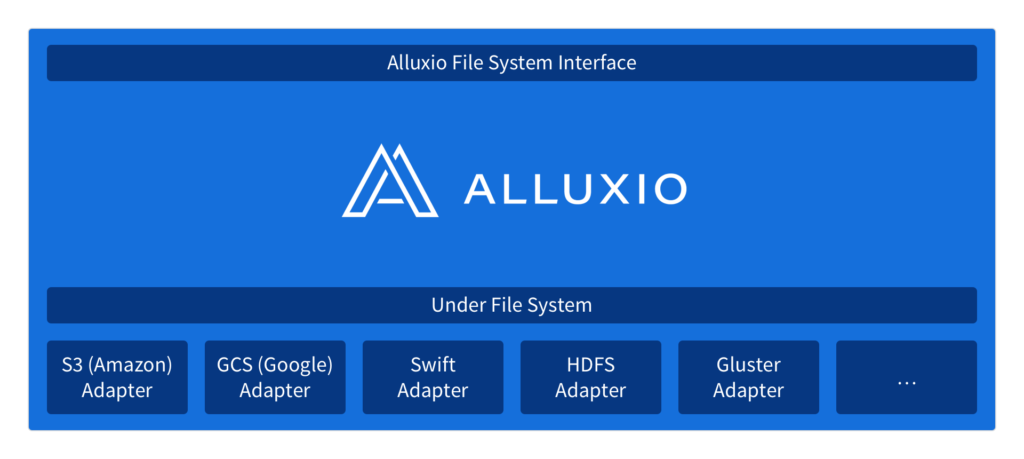
However, Alluxio’s unified namespace is not just an interface; it is also a set of adapters that make it possible to use the same interface to access many popular storage systems. In addition, accessing data through Alluxio leverages Alluxio’s memory-centric data management layer, enabling significant performance benefits.
Similar to how a personal computer maps different local paths to different devices, Alluxio maps different Alluxio paths to different underlying storage systems. The mapping is dynamic and Alluxio provides an API for creating and removing these mappings and transparently surfacing objects from the underlying storage system in Alluxio.
Examples
In this section, we walk through a couple examples to illustrate how Alluxio’s unified namespace works in the real world.
Working With Multiple Storage Systems
In this example, we illustrate how Alluxio’s unified namespace enables applications to use one API to interact with multiple different storage systems at the same time.
For the sake of this example, we assume that the application is written in Java and is sharing data between HDFS and S3.
The following logic illustrates how Alluxio can be used to read data from HDFS, process it, and then write the results to S3:
package io;
import
alluxio.client.file.FileInStream;
import
alluxio.client.file.FileOutStream;
import
alluxio.client.file.FileSystem;
// mount
HDFS and S3 to Alluxio
FileSystem fileSystem = FileSystem.Factory.get();
fileSystem.mount("/mnt/hdfs", "hdfs://...");
fileSystem.mount("/mnt/s3", "s3n://...");
// read
data from HDFS
AlluxioURI inputUri = new AlluxioURI("/mnt/hdfs/input.data");
FileInStream is = fileSystem.openFile(inputUri);
... // read
data
is.close();
... // perform computation
// write data to S3
AlluxioURI outputUri = new AlluxioURI("/mnt/s3/output.data");
FileOutStream os = fileSystem.createFile(outputUri);
... // write data
os.close();
A special case of the above example is the following logic, which can be used to copy data from one storage system to another.
package io;
import
alluxio.client.file.FileInStream;
import
alluxio.client.file.FileOutStream;
import
alluxio.client.file.FileSystem;
import
org.apache.commons.io.IOUtils;
// mount
HDFS and S3 to Alluxio
FileSystem fileSystem = FileSystem.Factory.get();
fileSystem.mount("/mnt/hdfs", "hdfs://...")
fileSystem.mount("/mnt/s3", "s3n://...")
// copy data from HDFS to S3
AlluxioURI inputUri = new AlluxioURI("/mnt/hdfs/input.data");
AlluxioURI outputUri = new AlluxioURI("/mnt/s3/output.data");
FileInStream is = fileSystem.openFile(inputUri);
FileOutStream os = fileSystem.createFile(outputUri);
IOUtils.copy(is, os);
is.close();
os.close();
A code-complete version of this example can be found here.
Porting an Application to a Different Storage System
In this example, we illustrate how using unified namespace greatly reduces the effort needed to port an application when the data the application accesses moves to a different storage system.
For the sake of this example, let us again assume that the application is written in Java and that the data it accesses moves from HDFS to S3.
Without Alluxio, the original application would use the HDFS API for read and writing the data:
package io;
import
org.apache.hadoop.conf.Configuration;
import
org.apache.hadoop.fs.FSDataInputStream;
import
org.apache.hadoop.fs.FSDataOutputStream;
import
org.apache.hadoop.fs.FileSystem;
import
org.apache.hadoop.fs.Path;
// reading data
Path path = new Path("hdfs://<host>:<port>/<path>");
FileSystem fileSystem = path.getFileSystem(new Configuration());
FSDataInputStream is = fileSystem.open(path);
... // read
data
is.close();
... // perform computation
// writing data
Path path = new Path("hdfs://<host>:<port>/<path>");
FileSystem fileSystem = path.getFileSystem(new Configuration());
FSDataOutputStream os = fileSystem.create(path);
... // write data
os.close();
When the data moves to S3, the application needs to use a different API, such as jets3t, to read and write the data:
package io;
import
org.jets3t.service.impl.rest.httpclient.RestS3Service;
import
org.jets3t.service.model.S3Object;
import
org.jets3t.service.security.AWSCredentials;
import
java.io.InputStream;
// reading data
RestS3Service client = new RestS3Service();
S3object object = client.getObject(<bucket>, <key>);
InputStream is = object.getDataInputStream();
... // read
data
is.close();
... // perform computation
// writing data
OutputStream os = new FileOutputStream();
... // write data
os.close();
InputStream is = new FileInputStream(<tmpFile>);
RestS3Service client = new RestS3Service(<credentials>);
S3Object object = new S3Object(<key>);
object.setDataInputStream(is);
client.putObject(<bucket>, object);
is.close();
In addition to the difference in the above logic, the logic for reading and writing data (not shown) needs to change as well to reflect the differences between HDFS API and S3 API.
In contrast to the above changes, with Alluxio, the application can use the following logic to access data from both HDFS and S3:
package io;
import
alluxio.client.file.FileInStream;
import
alluxio.client.file.FileOutStream;
import
alluxio.client.file.FileSystem;
// mount
a storage system (HDFS or S3) to Alluxio
FileSystem fileSystem = FileSystem.Factory.get();
fileSystem.mount("/mnt/data", <url>;)
// reading data
AlluxioURI uri = new AlluxioURI("/mnt/data/...");
FileInStream is = fileSystem.openFile(uri);
... // read
data
is.close();
... // perform computation
// writing data
AlluxioURI uri = new AlluxioURI("/mnt/data/...");
FileOutStream os = fileSystem.createFile(uri);
... // write data
os.close();
In particular, when data moves from HDFS to S3, the only part of the application that needs to be updated is the <url> to mount, which can be read from configuration or a command-line argument to avoid any application changes at all.
Conclusion
Alluxio’s unified namespace provides a layer of indirection that logically decouples the computation from data. Applications simply communicate with Alluxio while Alluxio handles the communication with the different underlying storage systems on applications’ behalf.
Alluxio supports many popular storage systems including S3, GCS, Swift, HDFS, OSS, GlusterFS, and NFS. Adding support for new storage systems is relatively straightforward — the existing adapters are less than a thousand lines of code each.
Please do not hesitate to contact us if you would like to propose or lead an integration. We welcome more integrations and look forward to hearing from you!
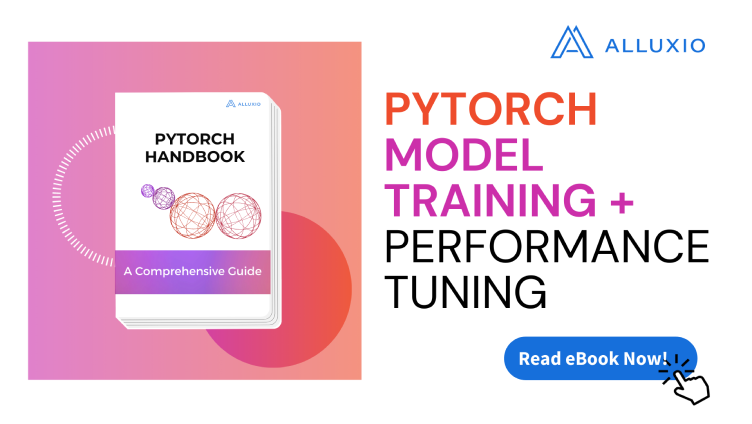
For business to not just survive — but to flourish — it’s become imperative to make decisions with near immediacy, continuously pivot strategy and tactics, and merge streams of inquiries into meaningful action. Executing requires high-frequency insights — the competitive advantage in today’s frenetic business landscape. Together with Alluxio, Inc., we enable businesses to gain the competitive advantage with faster time to insights with our integrated solution of Cray high-performance analytics platform and Alluxio’s memory-speed virtual storage system — Alluxio Enterprise Edition.
For businesses on the cusp of innovation and seeking that information advantage, Cray has fused supercomputing with an open, standards-based framework to deliver an industry first: the Cray® Urika®-GX agile analytics platform. This advanced platform has an unprecedented combination of versatility and speed to tackle the largest problems at super scale and uncover hidden patterns with a fast time to insight.
Alluxio provides a unified view of enterprise data that spans disparate storage systems, locations and clouds, allowing any big data compute framework to access stored data at memory speed. Alluxio Enterprise Edition gives Cray customers the ability to co-locate compute and data with memory-speed access to data while virtualizing across different storage systems under a unified namespace. Alluxio Enterprise Edition supercharges data storage and gives customers an easy-to-install integrated package, with an administrative interface as well as mission-critical features such as enterprise security, data replication and enterprise-grade support and professional services.

Alluxio proves its value in production scenarios where customers need to analyze vast amounts of data in real-time. It overcomes I/O limitations for running big data workloads that access remote storage systems and allows big data workloads to share data at memory speed for orders of magnitude higher performance in running jobs.
Benefits of the Urika-GX platform and Alluxio Enterprise Edition integration
Easy to deploy for access to multiple storage types
With this integration, you can deploy Alluxio in minutes and realize fast on-demand analytics by unified in-memory access to a multitude of data sources such as Ceph, HDFS, Amazon S3 and Google Cloud Storage. Choose between command-line and GUI-based installation to suit your preferences on any number of high-memory compute nodes on the Urika-GX platform.
The Urika-GX system is optimized for Apache Spark™ in-memory analytics, and combining that with Alluxio on Urika-GX gives you single data access gateway. You can expand this in-memory computation capabilities to other frameworks with Alluxio on the Urika-GX platform.
Unified view and management of all your data
Alluxio connects any compute framework, such as Spark or Presto, to disparate storage systems via a unified global file system namespace, and enables any application to interact with data stored on premise and in the cloud at memory speed. This eliminates data duplication and migration, as well as enables new workflows across data stored in any storage system, including cross-data center storage.
Efficient resource utilization
By decoupling compute from storage, Alluxio allows organizations to scale compute and storage resources independently. You can scale number of compute nodes with high-performance and reliable components and leave the job of data access to Alluxio on the Urika-GX platform, which utilizes Cray’s supercomputing network for node-to-node communication, thus ensuring effective “data-locality” even for remote data sources.
High-performance and predictable SLA
Alluxio is co-located with compute, and thereby provides memory-speed access to data, and orders of magnitude improvement in runtime for different types of jobs. The Urika-GX system, with its dense memory hierarchy, enables Alluxio to cache large amounts of multiple data types and sources to enable fast data access, high computational performance and predictable SLAs.
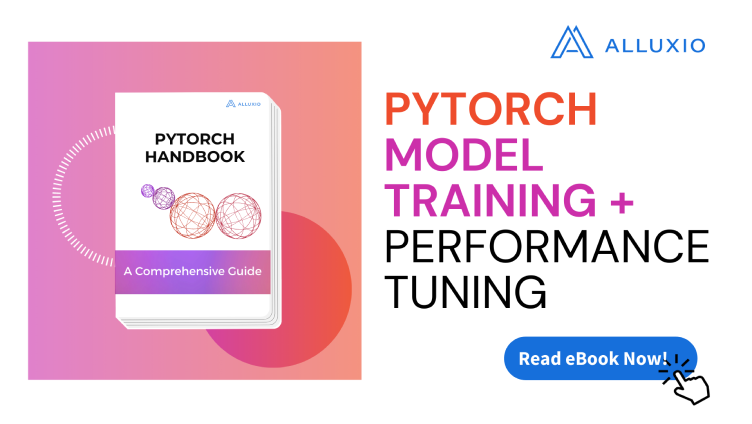
Introduction
Many organizations deploy Alluxio together with Spark for performance gains and data manageability benefits. Qunar recently deployed Alluxio in production, and their Spark streaming jobs sped up by 15x on average and up to 300x during peak times. They noticed that some Spark jobs would slow down or would not finish, but with Alluxio, those jobs could finish quickly. In this blog post, we investigate how Alluxio helps Spark be more effective. Alluxio increases performance of Spark jobs, helps Spark jobs perform more predictably, and enables multiple Spark jobs to share the same data from memory. Previously, we investigated how Alluxio is used for Spark RDDs. In this article, we investigate how to effectively use Spark DataFrames with Alluxio.
Alluxio and Spark CachING
Storing Spark DataFrames in Alluxio memory is very simple, and only requires saving the DataFrame as a file to Alluxio. This is very simple with the Spark DataFrame write
API. DataFrames are commonly written as parquet files, with df.write.parquet()
. After the parquet is written to Alluxio, it can be read from memory by using sqlContext.read.parquet()
.
In order to understand how saving DataFrames to Alluxio compares with using Spark cache, we ran a few simple experiments. We used a single worker Amazon EC2 r3.2xlarge instance, with 61 GB of memory, and 8 cores. We used Spark 2.0.0 and Alluxio 1.2.0 with the default configurations. We ran both Spark and Alluxio in standalone mode on the node. For the experiment, we tried different ways of caching Spark DataFrames, and saving DataFrames in Alluxio, and measured how the various techniques affect performance. We also varied the size of the data to show how data size affects performance.
Saving DataFrames
Spark DataFrames can be “saved” or “cached” in Spark memory with the persist()
API. The persist()
API allows saving the DataFrame to different storage mediums. For the experiments, the following Spark storage levels are used:
MEMORY_ONLY
: stores Java objects in the Spark JVM memoryMEMORY_ONLY_SER
: stores serialized java objects in the Spark JVM memoryDISK_ONLY
: stores the data on the local disk
Here is an example of how to cache a DataFrame with the persist()
API:
df.persist(MEMORY_ONLY)
An alternative way to save DataFrames to memory is to write the DataFrame as files in Alluxio. Spark supports writing DataFrames to several different file formats, but for these experiments we write DataFrames as parquet files. Here is an example of how to write a DataFrame to Alluxio memory:
df.write.parquet(alluxioFile)
Querying “saved” DataFrames in Alluxio
After DataFrames are saved, either in Spark or Alluxio, applications can read them to computations. In our experiments, we created a sample DataFrame with 2 float columns, and the computation was a sum on both columns.
When the DataFrame is stored in Alluxio, to read the data in Spark is as simple as reading the file from Alluxio. Here is an example of reading our sample DataFrame in Alluxio.
df
= sqlContext.read.parquet(alluxioFile)
df.agg(sum("s1"), sum("s2")).show()
We performed this aggregation on the DataFrame from Alluxio parquet files, and from various Spark persist storage levels, and we measured the time it took for the aggregation. The figure below shows the completion times for the aggregations.

The figure shows that performing the aggregation on the DataFrame read from the Alluxio parquet file results in very predictable and stable performance. However, when reading DataFrames from the Spark cache, the performance is high for small data sizes, but larger data sizes significantly hurts the performance. For the various Spark storage levels, after about 20GB of input data, the aggregation slows down and increases significantly.
With Alluxio memory, the DataFrame aggregation performance is slightly slower than with Spark memory for smaller data sizes, but as the data size grows, reading from Alluxio performance significantly better as it scales linearly with the data size. Since the performance scales linearly, applications can process larger data sizes at memory speeds with Alluxio.
Sharing “saved” DataFrames with Alluxio
Alluxio also enables the ability to share data in-memory, even across different Spark jobs. After a file is written to Alluxio, that same file can be shared across different jobs, contexts, and even frameworks, via Alluxio’s memory. Therefore, if a DataFrame in Alluxio is frequently accessed by many applications, all the applications can read the data from the in-memory Alluxio file, and do not have to recompute it or fetch it from an external source.
To demonstrate the in-memory sharing benefits of Alluxio, we computed the same DataFrame aggregation in the same environment as described above. With the 50GB data size, we ran the aggregation in a separate Spark application, and measured the time it took to perform the computation. Without Alluxio, the Spark application must read the data from the source, which is the local SSD in this experiment. However, when using Spark with Alluxio, reading the data means reading it from Alluxio memory. Below are the results of the completion time for the aggregation.
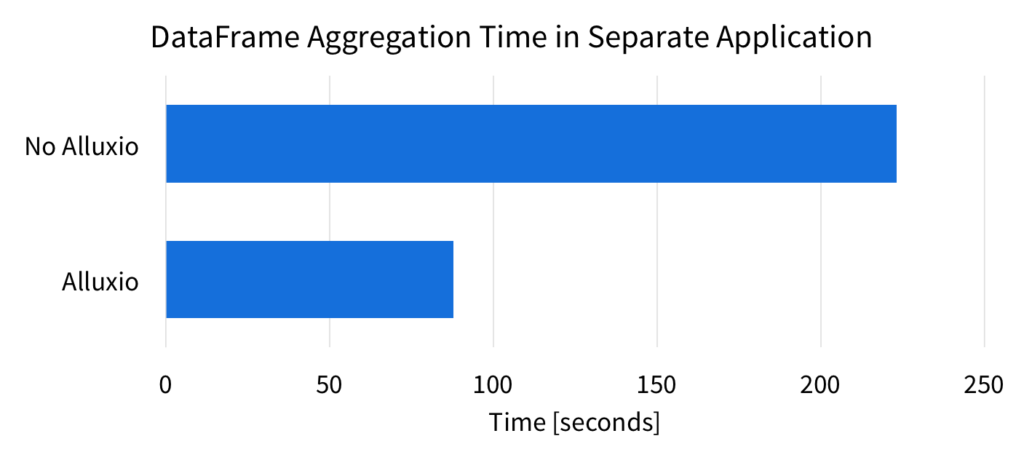
Without Alluxio, Spark has to read the data from the source again (local SSD). Reading from Alluxio is faster since the data is read from memory. With Alluxio, the aggregation is over 2.5x faster.
In the previous experiment, the source of the data was the local SSD. However, if the source of the DataFrame is slower or less predictable, the benefits of Alluxio is more significant. For example, Amazon S3 is a popular system for storing large amounts of data. Below are the results for when the source of the DataFrame is from Amazon S3.

The figure shows the average aggregation completion time over 7 runs. The error bars in the figure represent the min and max range of the completion times. These results clearly show, that Alluxio significantly improves the average performance of the computation. This is because with Alluxio, Spark can read the DataFrame directly from Alluxio memory, instead of fetching the data from S3 again. On average, Alluxio speeds up the DataFrame computation over 10x.
Since the source of the data is Amazon S3, Spark without Alluxio has to fetch the data over the network, and this can result in unpredictable performance. This unstable performance is evident from the error bars of the figure. Without Alluxio, the Spark job completion times widely vary, by over 1100 seconds. With Alluxio, the completion times only vary by 10 seconds. Alluxio reduces the unpredictability by over 100x!
Because of the S3 network unpredictability, the slowest Spark run without Alluxio can take as long as over 1700 seconds, almost twice as slow as the average. On the other hand, the slowest Spark run with Alluxio is around 6 second slower than the average time. By considering the slowest runs, Alluxio speeds up the DataFrame aggregation by over 17x.
Conclusion
Alluxio helps Spark be more effective by enabling several benefits. This blog demonstrates how to use Alluxio with Spark DataFrames, and presents performance evaluations of the benefits.
- Alluxio can keep larger data sizes in memory to speed up Spark applications
- Alluxio enable sharing of data in-memory
- Alluxio provides stable and predictable performance
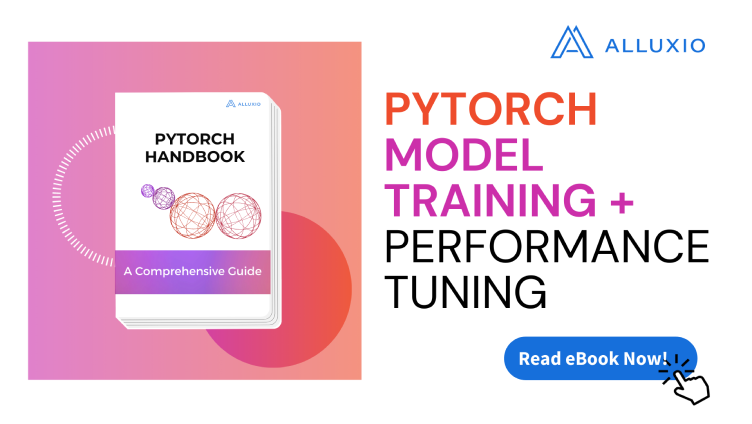
A Fortune 50 technology company that serves over 1 billion users successfully implemented Alluxio to achieve a hybrid cloud strategy, become multi-cloud ready, cut costs, and boost agility.
This case study highlights:
- This tech giant’s cloud journey to modernize its data platform and the challenges it faces
- Why this company chose Alluxio to achieve hybrid and multi-cloud
- The architecture and best practices of hybrid and multi-cloud data platform architecture with Alluxio
- How Alluxio helps the company standardize the data stack and access data anywhere across all environments

.avif)
AI Platform and Data Infrastructure teams rely on Alluxio Data Acceleration Platform to boost the performance of data-intensive AI workloads, empower ML engineers to build models faster, and lower infrastructure costs.
With high-performance distributed cache architecture as its core, the Alluxio Data Acceleration Platform decouples storage capacity from storage performance, enabling you to more efficiently and cost-effectively grow storage capacity without worrying about performance.
- Data Acceleration
- Simplicity at Scale
- Architected for AI Workload Portability
- Lower Infrastructure Costs
In this datasheet, you will learn how Alluxio helps eliminate data loading bottlenecks and maximize GPU utilization for your AI workloads.
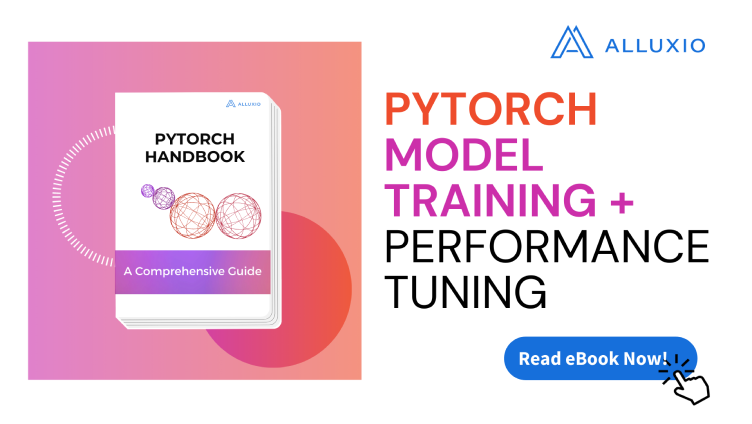
Apache Spark is an open source computation framework that supports a wide range of analytics — ETL, SQL queries, machine learning, and streaming computation. Spark’s in-memory data model and fast processing make it widely adopted by data-driven organizations.
For a global data platform, the following factors often result in slow time-to-value, high costs, and reduced agility:
- Today, data resides in silos, including data lakes, data warehouses, and object stores, whether on-premises, in the cloud, or spanning multiple geographic locations. It is challenging to find a solution that unifies data from many sources and serves it efficiently to Spark.
- An end-to-end data pipeline requires Spark to be used along with other compute frameworks like Presto, TensorFlow, etc., which requires a holistic approach to design the architecture of the data platform. Furthermore, organizations are stuck with legacy data platforms built in the pre-cloud era and lack cloud-native capabilities or require complex migrations to the cloud.
Are you seeking architectural innovation to address these challenges? Alluxio is here to help. Originating from the UC Berkeley AMPLab – the same lab as Spark, Alluxio is an open source data orchestration platform that bridges the gap between compute and storage. Alluxio empowers Spark by unifying data silos, providing data sharing across compute frameworks, and seamlessly migrating data across different environments.
By bringing Alluxio together with Spark, you can modernize your data platform in a scalable, agile, and cost-effective way. In this post, we provide an overview of the Spark + Alluxio stack. We explain the architecture, discuss real-world examples, describe deployment models, and showcase performance and cost benchmarking.
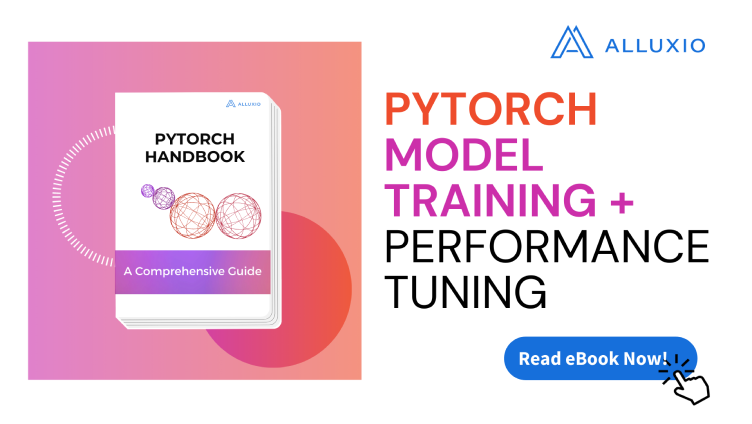
This whitepaper introduces how to speed up end-to-end distributed training in the cloud using Alluxio to accelerate data access. With the help of Alluxio, loading data from cloud storage, training and caching data can be done in a transparent and distributed way as a part of the training process. This whitepaper also demonstrates how to set up and benchmark the end-to-end performance of the training process, along with a comparison of other popular approaches.
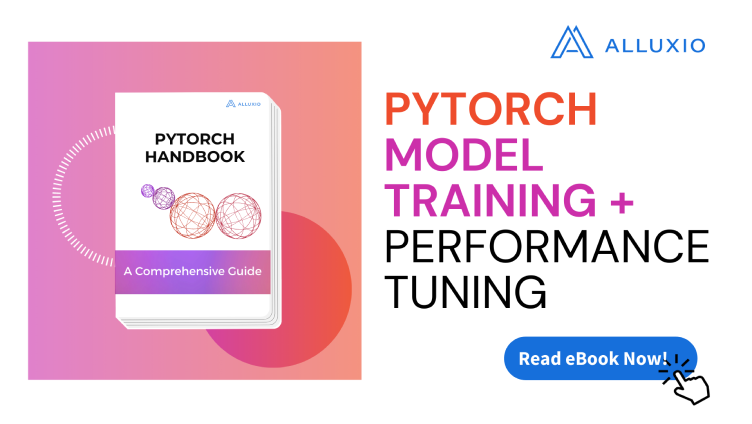
Presto (PrestoDB and Trino) is a popular choice for organizations to run interactive analytic queries on multiple data sources at a large scale. Positioned as SQL-on-Everything, Presto is designed to be a storage-independent engine that queries against any disparate data sources anywhere.
Many organizations are constantly modernizing their data platforms and developing scalable solutions to fit their existing and future needs. Although Presto is proven to have massive data processing capabilities, it is not optimized while dealing with data access across the pipeline. As a result, data platform engineers still have to find extra solutions to eliminate data redundancy, error-prone, slow and inconsistent performance, and high costs.
To solve these challenges, an innovative architecture combining Presto with Alluxio together is recommended. Alluxio is a data orchestration platform that bridges computation frameworks and underlying storage systems. Presto and Alluxio working together enables a unified, robust, high-performance, low-latency, and cost-effective analytics architecture. This architecture not only benefits analytics but also all stages of the data pipeline, spanning ingestion, analytics, and modeling. It allows fast SQL queries across multiple storage systems on-prem, in public cloud, hybrid cloud, and multi-cloud environments.
Many companies have leveraged Alluxio to level up their current Presto platform, including Facebook, TikTok, Electronic Arts, Walmart, Tencent, Comcast, and more. They have gained significant benefits with Alluxio integrated into their Presto stack.
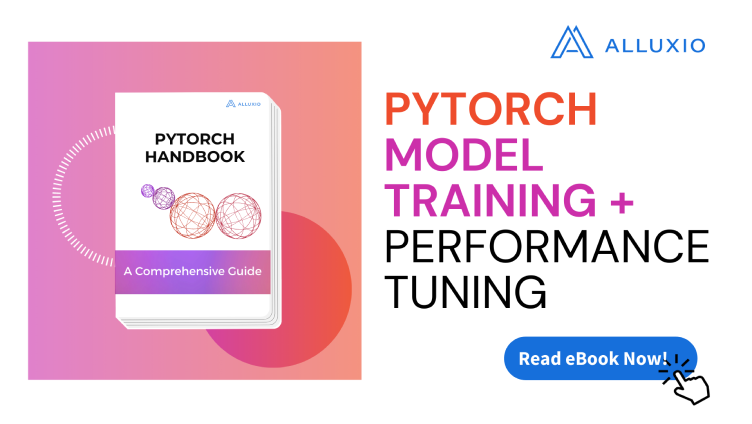
Alluxio started as a virtual distributed file system, a research project out of the AMPLab at U.C. Berkeley. Alluxio foresaw the need for agility when accessing large data stores separated from compute engines like Hadoop or Spark.
Fast forward several years and over a thousand committers later, and Alluxio has blossomed into the industry’s leading data orchestration platform for analytics and AI/ML. But as with any new type of technology, figuring out the best ways to use it depends on your data environment, computational workloads, issues, and goals.
So, after working with hundreds of organizations and fielding numerous inquiries, we thought it would be good to offer up a fresh take on the most popular use cases for Alluxio data orchestration.
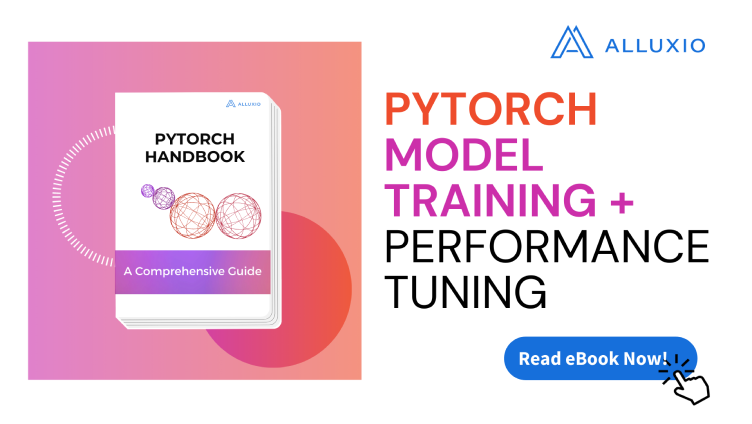
Large-scale analytics and AI/ML applications require efficient data access, with data increasingly distributed across multiple data stores in private data centers and clouds. Data platform teams also need the flexibility to introduce new data sources and move to new storage options with minimal changes or downtime for their applications. This paper delves further into what’s driving the need for–and what problems are solved with—an Alluxio data orchestration layer as part of a modern data platform.
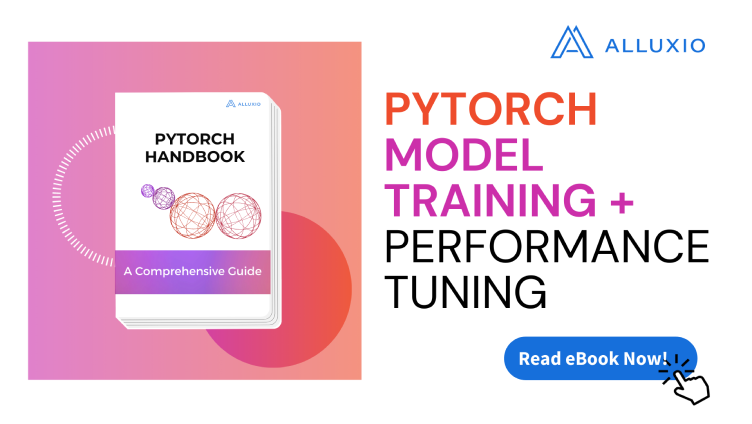
As ever more big data computations start to be in-memory, I/O throughput dominates the running times of many workloads. For distributed storage, the read throughput can be improved using caching, however, the write throughput is limited by both disk and network bandwidth due to data replication for fault-tolerance. This paper proposes a new file system architecture to enable frameworks to both read and write reliably at memory speed, by avoiding synchronous data replication on writes.
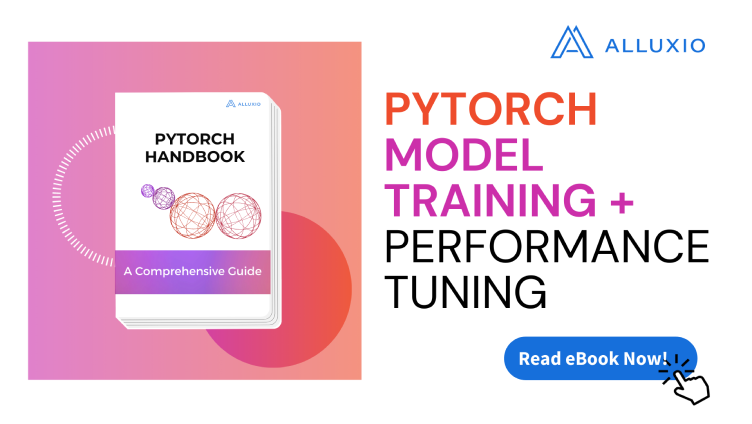
Tachyon is a distributed file system enabling reliable data sharing at memory speed across cluster computing frameworks. While caching today improves read workloads, writes are either network or disk bound, as replication is used for fault-tolerance. Tachyon eliminates this bottleneck by pushing lineage, a well-known technique, into the storage layer. The key challenge in making a long-running lineagebased storage system is timely data recovery in case of failures. Tachyon addresses this issue by introducing a checkpointing algorithm that guarantees bounded recovery cost and resource allocation strategies for recomputation under commonly used resource schedulers. Our evaluation shows that Tachyon outperforms in-memory HDFS by 110x for writes. It also improves the end-to-end latency of a realistic workflow by 4x. Tachyon is open source and is deployed at multiple companies.